일 | 월 | 화 | 수 | 목 | 금 | 토 |
---|---|---|---|---|---|---|
1 | ||||||
2 | 3 | 4 | 5 | 6 | 7 | 8 |
9 | 10 | 11 | 12 | 13 | 14 | 15 |
16 | 17 | 18 | 19 | 20 | 21 | 22 |
23 | 24 | 25 | 26 | 27 | 28 |
- 미지아
- k8s
- synology
- lubuntu
- 트랙포인트
- talos linux
- TRACKPOINT
- k0s
- 울트라나브
- 미니스튜디오
- Thunderbolt3
- 쿠버네티스
- Code-Server
- centos
- 스마트 디스플레이
- 탁상시계
- Rocky LInux
- 빨콩
- 시놀로지
- IoT
- VMM
- 원격
- Kubernetes
- ACASIS
- lenovo
- USB4.0
- 포토박스
- 외장SSD
- SSD인클로저
- 가상컴퓨터
- Today
- Total
테크믈리에의 리뷰 공간
[k0s Cluster 구축] 4. k0s 클러스터에서 Nvidia GPU 사용하기 본문
k0s 클러스터에서 Nvidia GPU를 사용하기 위해서는 Rancher 시리즈에서 했던 것과 마찬가지로 Containerd 환경 설정을 변경하고 Node Feature Discovery를 설치한 다음 GPU Operator를 설치하는 과정이 필요하다.
우선, Nvidia GPU를 가상 컨테이너에서 접근하여 사용하기 위한 전제 조건은 Nvidia GPU Driver가 설치되어 있고 Nouveau 드라이버가 꺼져 있으며 Nvidia Container Toolkit이 설치되어 있을 것인데, 이 시리즈의 1번 글인 Ansible을 통한 기초 세팅을 따라했다면 이미 해당 전제 조건은 충족되어 있을 것이다.
k0s Containerd 설정파일 변경
제일 먼저 해줄 것은 k0s의 Containerd 설정파일을 변경하여 Containerd의 기본 Runtime으로 Nvidia-Container-Runtime을 지정하는 것이다.
보다 자세한 k0s의 Runtime 설정 방법은 하단 Reference 항목을 참고하도록 하자.
제일 먼저 할 것은 우선 동작 중인 클러스터를 잠시 멈춰주는 것이다.
# 루트 권한
su
# 각 k0s worker 노드에 접속하여 해당 노드 종료
# 만약 --enable-worker 옵션을 줬다면 마스터에서도 종료한다
k0s stop
그 다음으로는 각 GPU 장착된 워커노드에서 /etc/k0s/containerd.d/nvidia.toml 파일을 생성하여 아래 내용을 붙여넣는다.
[plugins."io.containerd.grpc.v1.cri".containerd.runtimes.nvidia]
privileged_without_host_devices = false
runtime_engine = ""
runtime_root = ""
runtime_type = "io.containerd.runc.v2"
[plugins."io.containerd.grpc.v1.cri".containerd.runtimes.nvidia.options]
BinaryName = "/usr/bin/nvidia-container-runtime"
붙여 넣고 k0s start를 통하여 각 노드를 다시 실행해준다면 해당 설정이 적용될 것이다.
적용 이후 다시 k0s 클러스터를 멈춰주고 /etc/k0s/containerd.toml 파일을 열어보도록 하자.
파일을 열어보면 위와 같이 정의가 되어있을텐데, 내용은 결국 /run/k0s/containerd-cri.toml 파일을 참조한다는 것 뿐이다.
이제 우리는 수동으로 설정을 적용해야하기 때문에 최상단의 #k0s_managed=true를 false로 변경해주도록 하자.
그 다음 /run/k0s/containerd-cri.toml 파일을 열어서 중간에 nvidia.toml 파일에 적어두었던 내용이 삽입되어 있는지 확인해주도록 하자.
정상적으로 내용이 삽입되어 있다면 이번에는 default_runtime_name을 검색한 다음 값을 "nvidia"로 변경해주도록 하자.
설정을 변경하였다면 값을 저장하고 다시 k0s start를 통해 클러스터를 활성화하도록 하자.
만약 일일히 모든 GPU 워커 노드에서 이 작업을 하기 귀찮다면, 첫 노드에서만 위 작업을 진행한 다음 /run/k0s/containerd-cri.toml 파일 내용을 전체 복사하여 /etc/k0s/containerd.toml 파일의 주석 아래 부분을 전부 지워버린 다음 덮어씌워버리자.
그 다음 /etc/k0s/containerd.toml 파일 내용을 전체 복사하여 굳이 nvidia.toml 파일 생성 없이 바로 나머지 GPU 워커 노드의 /etc/k0s/containerd.toml 파일에 똑같이 덮어 씌운 다음 k0s를 재시작해주면 정상적으로 동작할 것이다.
아래는 본인이 사용한 /etc/k0s/containerd.toml 파일 내용이다.
# k0s_managed=false
# This is a placeholder configuration for k0s managed containerD.
# If you wish to override the config, remove the first line and replace this file with your custom configuration.
# For reference see https://github.com/containerd/containerd/blob/main/docs/man/containerd-config.toml.5.md
version = 2
[plugins]
[plugins."io.containerd.grpc.v1.cri"]
cdi_spec_dirs = ["/etc/cdi", "/var/run/cdi"]
device_ownership_from_security_context = false
disable_apparmor = false
disable_cgroup = false
disable_hugetlb_controller = true
disable_proc_mount = false
disable_tcp_service = true
drain_exec_sync_io_timeout = "0s"
enable_cdi = false
enable_selinux = false
enable_tls_streaming = false
enable_unprivileged_icmp = false
enable_unprivileged_ports = false
ignore_image_defined_volumes = false
image_pull_progress_timeout = "1m0s"
max_concurrent_downloads = 3
max_container_log_line_size = 16384
netns_mounts_under_state_dir = false
restrict_oom_score_adj = false
sandbox_image = "registry.k8s.io/pause:3.8"
selinux_category_range = 1024
stats_collect_period = 10
stream_idle_timeout = "4h0m0s"
stream_server_address = "127.0.0.1"
stream_server_port = "0"
systemd_cgroup = false
tolerate_missing_hugetlb_controller = true
unset_seccomp_profile = ""
[plugins."io.containerd.grpc.v1.cri".cni]
bin_dir = "/opt/cni/bin"
conf_dir = "/etc/cni/net.d"
conf_template = ""
ip_pref = ""
max_conf_num = 1
setup_serially = false
[plugins."io.containerd.grpc.v1.cri".containerd]
default_runtime_name = "nvidia"
disable_snapshot_annotations = true
discard_unpacked_layers = false
ignore_blockio_not_enabled_errors = false
ignore_rdt_not_enabled_errors = false
no_pivot = false
snapshotter = "overlayfs"
[plugins."io.containerd.grpc.v1.cri".containerd.default_runtime]
base_runtime_spec = ""
cni_conf_dir = ""
cni_max_conf_num = 0
container_annotations = []
pod_annotations = []
privileged_without_host_devices = false
privileged_without_host_devices_all_devices_allowed = false
runtime_engine = ""
runtime_path = ""
runtime_root = ""
runtime_type = ""
sandbox_mode = ""
snapshotter = ""
[plugins."io.containerd.grpc.v1.cri".containerd.default_runtime.options]
[plugins."io.containerd.grpc.v1.cri".containerd.runtimes]
[plugins."io.containerd.grpc.v1.cri".containerd.runtimes.nvidia]
privileged_without_host_devices = false
runtime_engine = ""
runtime_root = ""
runtime_type = "io.containerd.runc.v2"
[plugins."io.containerd.grpc.v1.cri".containerd.runtimes.nvidia.options]
BinaryName = "/usr/bin/nvidia-container-runtime"
[plugins."io.containerd.grpc.v1.cri".containerd.runtimes.runc]
base_runtime_spec = ""
cni_conf_dir = ""
cni_max_conf_num = 0
container_annotations = []
pod_annotations = []
privileged_without_host_devices = false
privileged_without_host_devices_all_devices_allowed = false
runtime_engine = ""
runtime_path = ""
runtime_root = ""
runtime_type = "io.containerd.runc.v2"
sandbox_mode = "podsandbox"
snapshotter = ""
[plugins."io.containerd.grpc.v1.cri".containerd.runtimes.runc.options]
BinaryName = ""
CriuImagePath = ""
CriuPath = ""
CriuWorkPath = ""
IoGid = 0
IoUid = 0
NoNewKeyring = false
NoPivotRoot = false
Root = ""
ShimCgroup = ""
SystemdCgroup = false
[plugins."io.containerd.grpc.v1.cri".containerd.untrusted_workload_runtime]
base_runtime_spec = ""
cni_conf_dir = ""
cni_max_conf_num = 0
container_annotations = []
pod_annotations = []
privileged_without_host_devices = false
privileged_without_host_devices_all_devices_allowed = false
runtime_engine = ""
runtime_path = ""
runtime_root = ""
runtime_type = ""
sandbox_mode = ""
snapshotter = ""
[plugins."io.containerd.grpc.v1.cri".containerd.untrusted_workload_runtime.options]
[plugins."io.containerd.grpc.v1.cri".image_decryption]
key_model = "node"
[plugins."io.containerd.grpc.v1.cri".registry]
config_path = ""
[plugins."io.containerd.grpc.v1.cri".registry.auths]
[plugins."io.containerd.grpc.v1.cri".registry.configs]
[plugins."io.containerd.grpc.v1.cri".registry.headers]
[plugins."io.containerd.grpc.v1.cri".registry.mirrors]
[plugins."io.containerd.grpc.v1.cri".x509_key_pair_streaming]
tls_cert_file = ""
tls_key_file = ""
Node Feature Discovery & GPU Operator
curl https://raw.githubusercontent.com/helm/helm/main/scripts/get-helm-3 | bash
우선은 Node Feature Discovery부터 올리도록 하자.
helm repo add nfd https://kubernetes-sigs.github.io/node-feature-discovery/charts
helm repo update
helm install nfd/node-feature-discovery --namespace node-feature-discovery --create-namespace --generate-name
설치 후 정상적으로 NFD 파드들이 전부 생성될 때까지 기다린 다음 GPU Operator를 올리도록 하자.
우선은 GPU Operator의 Repository부터 등록하자.
helm repo add nvidia https://nvidia.github.io/gpu-operator && helm repo update
그 다음에는 k0s 값에 맞도록 values.yaml을 수정해서 설치해야하는데, 우선 gpu-operator의 전체 values.yaml을 확인하는 방법은 다음과 같다.
helm show values nvidia/gpu-operator >> gpu-operator-values.yaml
해당 values 내용 중 우리가 변경해야할 요소는 다음과 같다.
- operator.defaultRuntime = containerd
- driver.enabled = false
- toolkit.enabled = false
- toolkit.env[0].name = CONTAINERD_CONFIG, toolkit.env[0].value = "/etc/k0s/containerd.toml"
- toolkit.env[1].name = CONTAINERD_SOCKET, toolkit.env[1].value = "/run/k0s/containerd.sock"
- toolkit.env[2].name = CONTAINERD_RUNTIME_CLASS, toolkit.env[2].value = "nvidia"
- toolkit.env[3].name = CONTAINERD_SET_AS_DEFAULT, toolkit.env[3].value = "true"
위의 사진과 내용을 확인하여 valeus 파일을 수정해주도록 하자.
helm install gpu-operator -n gpu-operator --create-namespace nvidia/gpu-operator -f gpu-operator-values.yaml
그 다음에 위 명령어를 사용하여 GPU Operator를 설치하여주도록 하자.
성공적으로 설치되었다면, 어느 정도 시간 뒤에 Node Labels를 확인하면 위와 같이 GPU 정보가 노출될 것이다.
보다 자세하게 검증하기 위해서는 우선 아래 내용을 gpu_test.yaml 이란 이름으로 저장해주도록 하자.
apiVersion: v1
kind: Pod
metadata:
name: ubuntu-18.04
spec:
containers:
- name: ubuntu
image: nvidia/cuda:11.1.1-devel-ubuntu20.04
command:
- "/bin/sleep"
- "604800"
resources:
limits:
cpu: "2"
memory: "8G"
nvidia.com/gpu: "1"
kubectl apply -f gpu_test.yaml
그 다음에는 위 명령어를 통하여 테스트용 Pod를 올리도록 한다.
Pod가 활성화 된 이후에는 아래 명령어를 통하여 테스트해볼 수 있다.
# Pod 접속
kubectl exec -it ubuntu-18.04 -- /bin/bash
# 접속 후에
nvidia-smi
만약 정상적으로 nvidia-smi 결과값이 나온다면 성공이다.
kubectl delete -f gpu_test.yaml
테스트가 끝난 후에는 위의 명령어로 Pod를 삭제하자.
Symlink 관련해서 오류가 나 제대로 설치되지 않는 상황이라면 gpu-operator-values.yaml에 다음 항목을 추가하도록 하자.
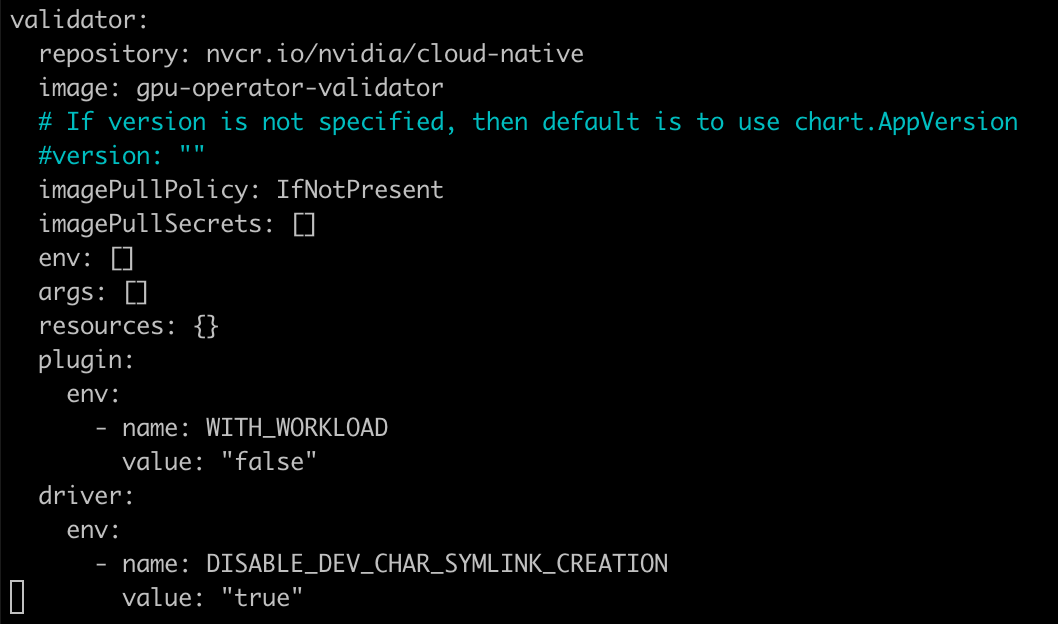
validator:
driver:
env:
- name: DISABLE_DEV_CHAR_SYMLINK_CREATION
value: "true"
Reference
https://docs.k0sproject.io/v1.28.4+k0s.0/runtime/
'프로그래밍|소프트웨어 > K0S' 카테고리의 다른 글
[k0s Cluster 구축] 5. k0s 클러스터에서 Rook Ceph Block Storage 사용하기 (0) | 2023.12.01 |
---|---|
[k0s Cluster 구축] 3. OpenLens의 기초 세팅법 (0) | 2023.11.23 |
[k0s Cluster 구축] 2. k0s 클러스터 구축 및 OpenLens 기초 사용법 (0) | 2023.11.22 |
[k0s Cluster 구축] 1. Ansible을 통한 기초 세팅 (0) | 2023.11.21 |
[k0s Cluster 구축] 0. Rocky Linux 8.5 설치 (0) | 2023.11.21 |